

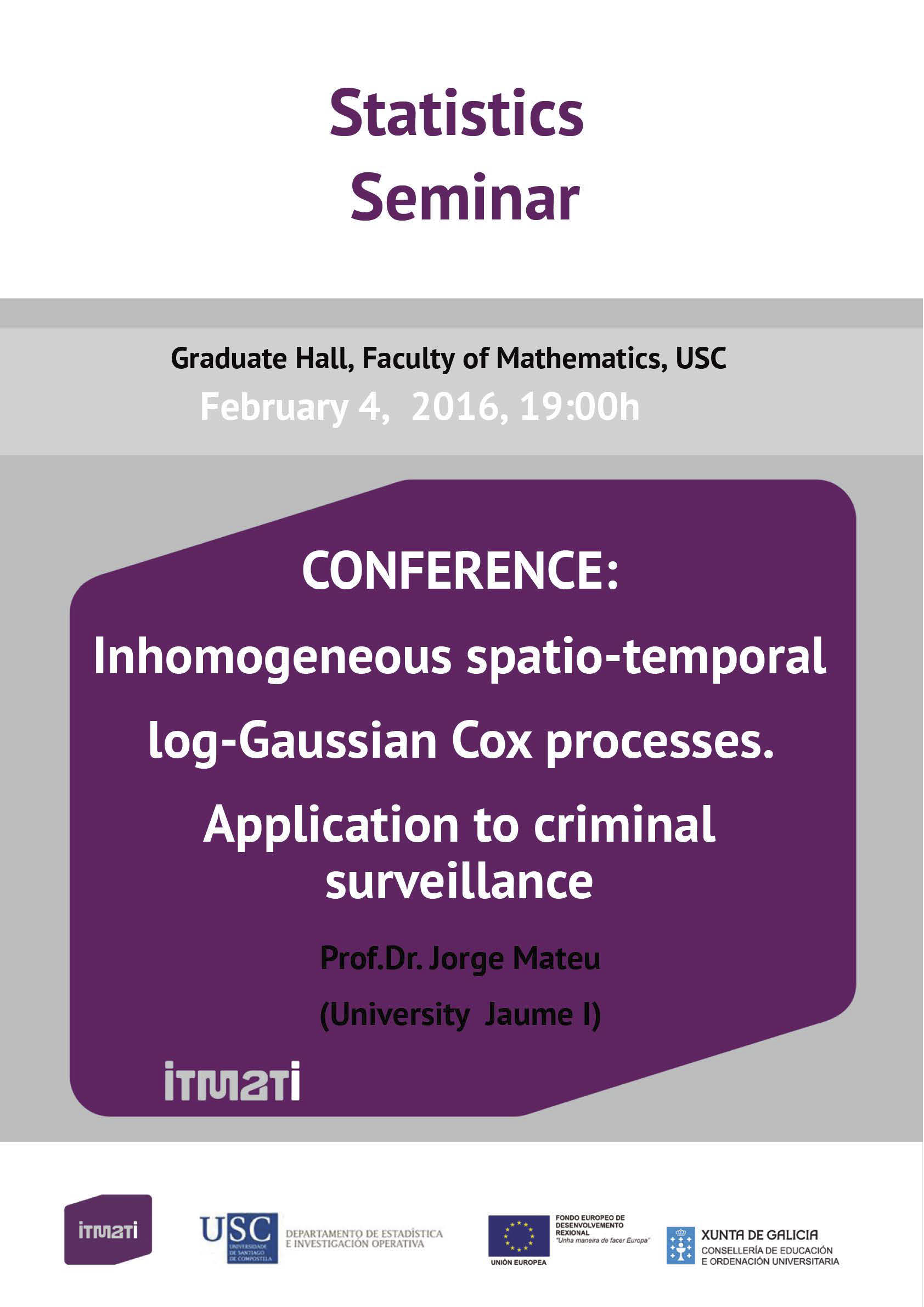
Next
February 4, 2016, Prof.
Dr. Jorge Mateu (Professor of Statistics and Operations Research, Department of Mathematics, Campus Riu Sec, Universitat Jaume I), will give the
Conference: Inhomogeneous spatio-temporal Cox log-Gaussian Processes . Application to criminal surveillance. The conference will be held at the Gradute Hall at the
Faculty fo Mathematics at University of Santiago de Compostela at
19:00 h.
Under the direction of Professor Jorge Mateu, the University Jaime I of Castellón, has developed a mathematical model sustaining by Pred-Crime, an algorithm to predict and prevent crimes, which aims to anticipate the future based on the crimes occurred in the past. It is a model of police intelligence that uses a huge database from crimes, which feeds a series of mathematical models and algorithms to generate risk maps to help to predict and prevent crime.
Crime, Gaussian random fields, Log-Gaussian Cox processes, Nonstationarity, Spatio-temporal point patterns, Surveillance
Surveillance systems have their origins in industrial applications, where the name refers to the routine collection and analysis of data for quality control purposes. More recently, the increasing need for early detection of disease outbreaks to inform prevention and control policies has given rise to an extensive literature on public health surveillance systems. Surveillance methods have been developed for other areas of application, for example, to detect acts of bioterrorism. Our motivation is from criminology, in particular prediction of crime events, and detection of emergent clusters in the spatio-temporal distribution of such crimes.
We propose a method for conducting likelihood-based inference for a class of nonstationary spatio-temporal log-Gaussian Cox processes. We derive an approximate conditional likelihood function for spatio-temporal log-Gaussian Cox processes. The method uses low-rank convolution-based models to capture the spatio-temporal correlation structure, to alleviate the computational burden involved in applying likelihood-based methods to full rank models.
We describe an application to a surveillance system to model the spatio-temporal distribution of crime events with the aim of predicting and detecting emergent spatio-temporal clusters of crime events.
-
DATE AND PLACE: : February 4, 2016, Graduate Hall, Faculty of Mathematics, USC, 19:00h